Seminario 22/17: Germán López Buenache (Universidad de Murcia) Factor models for large and incomplete data sets with unknown group structure
Información
- Ponente: Germán López Buenache (Universidad de Murcia)
- Fecha: 01/Dic/2022 - 12:00 horas
- Lugar: Seminario de Métodos Cuantitativos. Facultad de Economía y Empresa. Universidad de Murcia
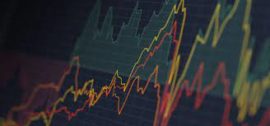
Most economic applications rely on a large number of time series, which typically have a remarkable clustering structure and they are available over different spans. To handle these databases, we combined the expectation–maximization (EM) algorithm outlined by Stock and Watson (JBES, 2002) and the estimation algorithm for large factor models with an unknown number of group structures and unknown membership described by Ando and Bai (JAE, 2016; JASA, 2017) . Several Monte Carlo experiments demonstrated the good performance of the proposed method at determining the correct number of clusters, providing the appropriate number of group-specific factors, identifying error-free group membership, and obtaining accurate estimates of unobserved missing data. In addition, we found that our proposed method performed substantially better than the standard EM algorithm when the data had a grouped factor structure. Using the Federal Reserve Economic Data FRED-QD, our method detected two distinct groups of macroeconomic indicators comprising the real activity indicators and nominal indicators. Thus, we demonstrated the usefulness of our group-specific factor model for studies of business cycle chronology and for forecasting purposes.